Introduction
In today’s dynamic financial landscape, data science has become a cornerstone of the FinTech and banking industries. It has emerged as the driving force behind informed decision-making, benefiting both customers and the financial industry as a whole. Credit bureaus like TransUnion help in this data-driven decision-making by employing techniques such as credit scoring and customer segmentation. This has led to a rise in developing and implementing machine learning models in this domain.
In this article, we delve into the pivotal role data science plays in shaping the world of finance. From the fundamental significance of credit scoring to the intricacies of data governance and the transformative power of customer segmentation, this exploration highlights how data science empowers financial institutions to make data-driven decisions.
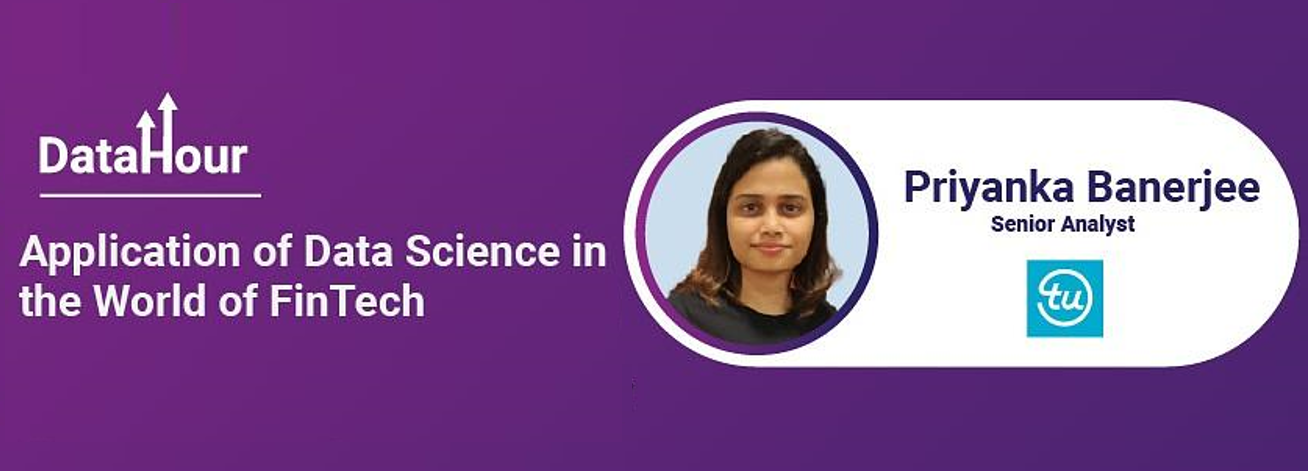
Learning Objectives:
- Understand the role and importance of credit scoring in FinTech.
- Learn about data governance and its significance in securing financial data.
- Discover how customer segmentation influences financial decision-making.
Credit Scoring and Financial Data Analysis in FinTech
Credit scoring is a fundamental cornerstone of the FinTech landscape. The credit score is what banks rely on to assess your eligibility for loans and an array of financial products. Think of it as a numerical gauge of your financial well-being. But it’s not a simple number; it’s a complex calculation influenced by many factors, including age, income, assets, etc.
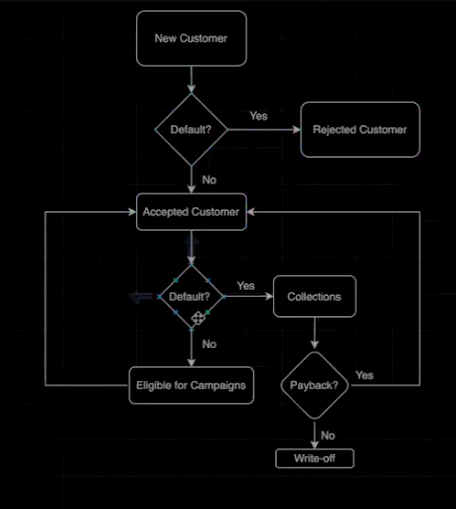
In the realm of FinTech, this credit score assumes a pivotal role in evaluating whether a customer is likely to default on a loan or credit card. Customers with a history of defaults may find themselves in the undesirable “rejected” category. In stark contrast, those boasting a favorable credit score gain access to various financial products. This finely tuned process hinges on extensive data analysis and predictive modeling, underpinning responsible lending practices.
Feature Selection and Model Deployment
With the vast amount of financial data available, it’s essential to streamline the information used to build predictive models. Feature selection is a key step in data analysis that helps narrow down the dataset to the most relevant variables. This process involves identifying highly correlated features and using statistical techniques to retain only the most informative ones. This not only improves model accuracy but also reduces computational costs and time.
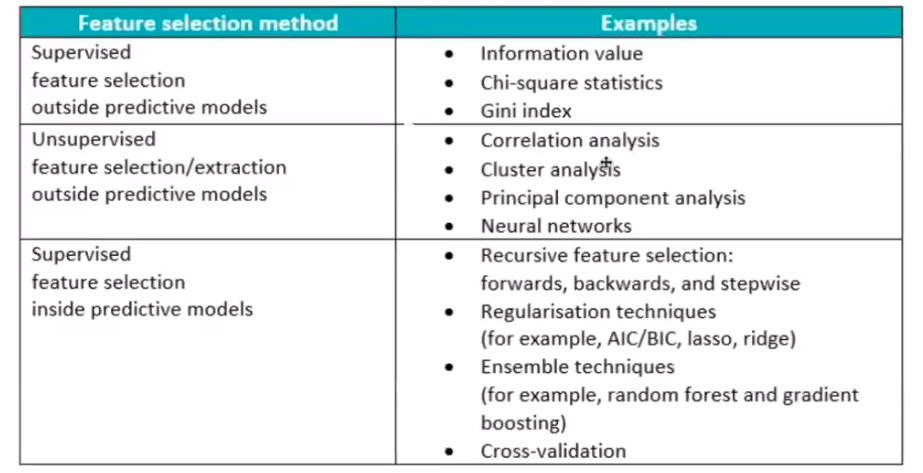
Once the features are selected, the predictive model is deployed to assess the creditworthiness of new customers. It’s a crucial step in minimizing the risk of loan defaults and ensuring responsible lending. The performance of these models is evaluated using metrics like the ROC curve, which measures the predictive ability of the classifier. In FinTech, an AUC (Area Under the Curve) of 0.75 or higher is the industry standard, indicating a robust model.
Model Evaluation and Metrics
Once a predictive model is crafted, it’s essential to assess its accuracy and reliability. In the realm of banking, decisions hinge on the ability of models to predict outcomes, such as whether a customer is likely to repay a loan. Traditional metrics like accuracy, precision, and recall play a role, but in-depth analysis goes beyond these basics.
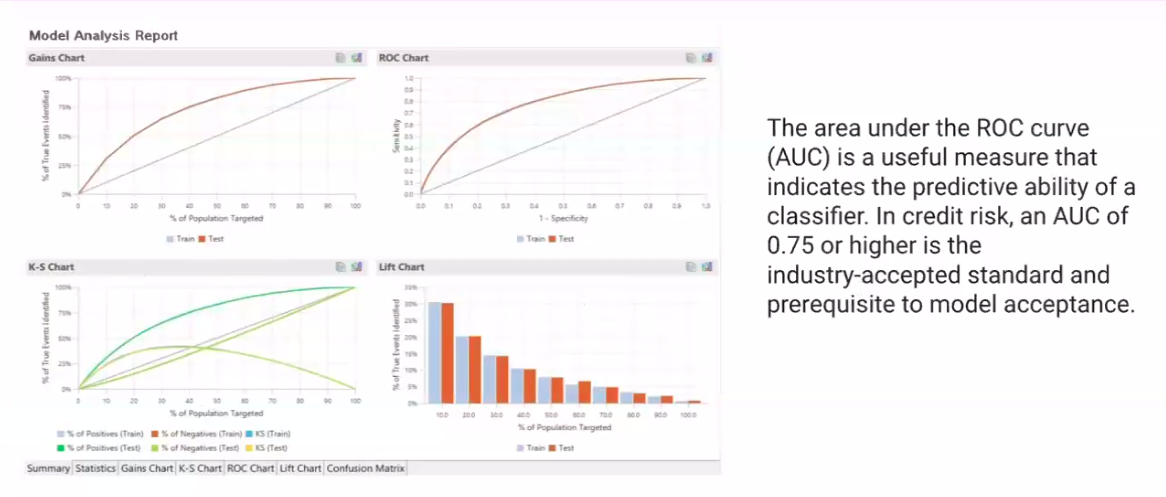
Two crucial metrics often used are the Kolmogorov-Smirnov (KS) test and the Gini coefficient. KS measures the separation between cumulative distribution functions of positive and negative classes. In essence, it tells us how well a model distinguishes between those who will buy a product (e.g., a credit card) and those who won’t. What’s notable is that KS doesn’t require a normal distribution, making it adaptable to various scenarios.
On the other hand, the Gini coefficient assesses the area between the Receiver Operating Characteristic (ROC) curve and the baseline. It dives deeper into the ROC curve, providing insights into the classifier’s performance. These metrics are vital when targeting specific customer segments, as understanding their behavior is pivotal in financial decision-making.
Model Monitoring
Model monitoring is an ongoing process, especially in the fast-paced world of fintech. Models built in the past can quickly become outdated due to evolving customer behavior, market dynamics, and regulatory changes. This is where monitoring steps in.
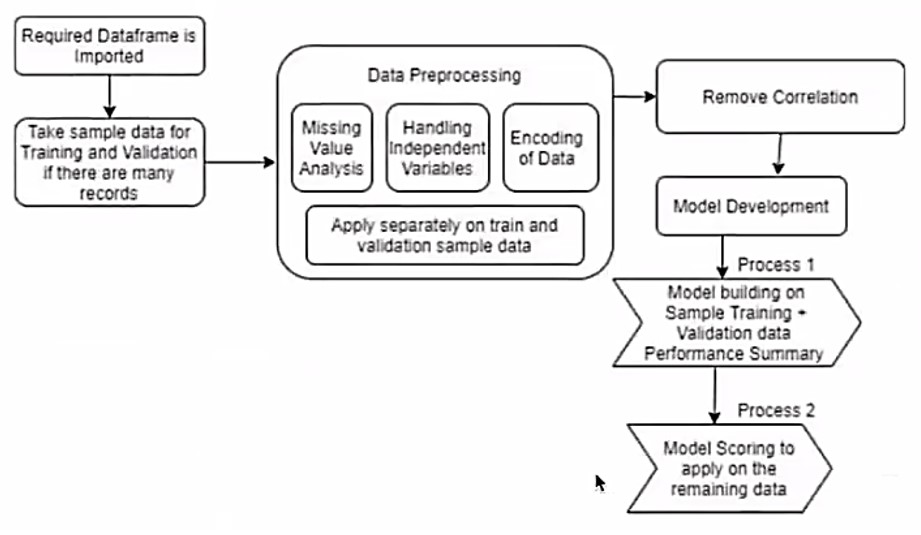
Regularly, metrics like KS and Gini are calculated on current data to compare against those from the development phase. The difference between these metrics serves as an indicator of how well the model is performing. Threshold values, often determined by business considerations, help decide if the model requires intervention. For instance, if the discrepancy exceeds 10% or 20%, a closer look is warranted.
In case of such significant discrepancies, there are two methods by which the model’s accuracy is tweaked: recalibration and redevelopment.
- Recalibration: Adjusting an existing model without changing its core features to fine-tune performance quickly.
- Redevelopment: Starting from scratch or making significant changes to both the model and features.
Recalibration is easier to do, and it fixes the issue in most cases. Redevelopment, on the other hand, is a more time-consuming process. This is because it often requires stakeholder approvals, used when fundamental improvements are needed.
Customer Segmentation and Marketing Campaigns
Marketing campaigns in the financial sector aren’t just about selling products; they’re about targeting the right customers. For example, when launching a co-brand card like the ICICI Bank Amazon credit card, the bank needs to find the right set of customers to market it to. By analyzing customer segments and their shopping behavior, banks can identify potential customers who might benefit from these cards. This targeted approach maximizes the effectiveness of marketing efforts and, in turn, increases revenue.
Customer segmentation is a potent tool for marketing in the fintech and banking sectors. By dividing customers into distinct groups based on behavior, financial institutions can personalize their offerings and marketing strategies. For instance, understanding that customers in a certain income bracket are more likely to default on loans can lead to targeted risk mitigation measures. Segmentation can also help identify opportunities for upselling or cross-selling products.
Data Governance: A Pillar of Trust
Data governance is the unsung hero in the fintech world. It ensures that data is handled, stored, and analyzed securely, adhering to strict regulations. As financial institutions collaborate with various data providers and handle sensitive customer information, maintaining data integrity is paramount. Data governance encompasses data ownership, policies, and quality. These aspects are subject to frequent changes driven by regulatory updates. Collaboration between banks, credit bureaus like TransUnion, and regulatory bodies like the RBI or Federal Reserve is crucial to maintaining data security and compliance.
TransUnion: Enabling Data-Driven Decision-Making
Credit bureaus like TransUnion play a pivotal role in the fintech ecosystem. They gather data from multiple banks and financial institutions, providing a comprehensive view of an individual’s financial history. This data is used to calculate credit scores and assess creditworthiness. TransUnion employs data clustering techniques to categorize individuals based on their financial behavior. For instance, owning a car might place you in a different cluster than someone with similar income but different financial commitments. These insights are invaluable for banks looking to offer tailored financial products.
Conclusion
In conclusion, data science stands at the forefront of the financial technology (FinTech) revolution, driving critical decisions and shaping the industry’s future. From credit scoring to model evaluation, data governance to customer segmentation, its influence is pervasive and transformative. The collaboration between financial institutions, credit bureaus like TransUnion, and regulatory bodies underscores the importance of data integrity and security in this dynamic landscape. As we advance in technology, data science will continue to empower banks and FinTech companies, leading to data-driven decisions that benefit both customers and the financial industry as a whole.
Key Takeaways:
- Credit scoring is a numerical representation of financial health that plays a vital role in FinTech.
- It influences lending decisions, enabling responsible lending practices based on extensive data analysis and predictive modeling.
- Efficient feature selection streamlines data for predictive models, enhancing accuracy while reducing computational costs.
- Model deployment is essential for assessing creditworthiness, ensuring responsible lending, and improving financial decision-making.
- Customer segmentation unlocks opportunities for personalized offerings and marketing strategies, benefiting both customers and financial institutions.
Frequently Asked Questions
Ans. Credit scoring is crucial in FinTech because it assesses an individual’s creditworthiness, enabling responsible lending practices. It helps banks and financial institutions make informed decisions about granting loans and other financial products.
Ans. Data governance in FinTech ensures secure handling, storage, and analysis of sensitive financial data, adhering to strict regulations. It is essential for maintaining data integrity, security, and compliance in a collaborative ecosystem.
Ans. Customer segmentation divides customers into distinct groups based on behavior, allowing financial institutions to personalize offerings and marketing strategies. It helps in risk mitigation, identifying upselling opportunities, and delivering tailored financial products to different customer segments.
About the Author: Priyanka Banerjee
Meet Priyanka, a seasoned Senior Analyst at TransUnion with a passion for leveraging data to drive impactful insights. Her expertise spans diverse domains, with a focus on Data Analysis, Machine Learning, Data Manipulation, Data Visualization, and Natural Language Processing. In her current role at TransUnion, she explores the various applications of data science in the world of FinTech and banking.
DataHour Page: https://community.analyticsvidhya.com/c/datahour/datahour-application-of-data-science-in-the-world-of-fintech
LinkedIn: https://www.linkedin.com/in/priyanka-banerjee-3005/